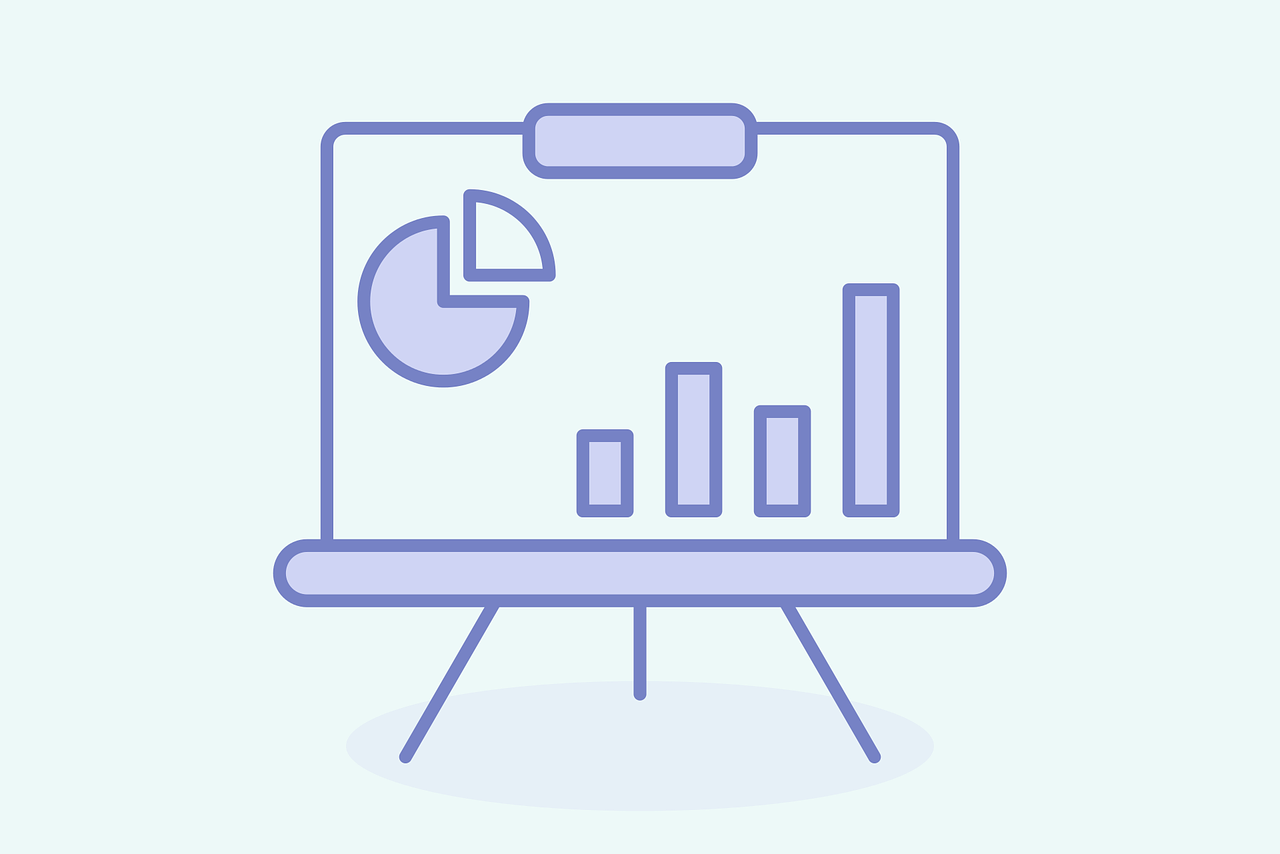
A Bayesian approach to explore and exploit molecular free-energy landscapes
Speaker: Eline Kempkes
Abstract:
To fully understand molecular processes, it is essential to know their free-energy landscape. However, determining this free-energy landscape exactly along a chosen pathway is usually very challenging, since many systems exhibit high free-energy barriers, which correspond to rare events. As a result, standard MD simulations cannot sample the entire configuration space, preventing full exploration of the free-energy landscape and pathways. To address this, several enhanced sampling methods have been introduced, such as umbrella sampling, steered MD, metadynamics, and others. In particular, umbrella sampling samples a specific region of the collective variable by adding a parabolic potential to the system, which provides a free-energy gradient estimation at that point. Sufficient sampling points can render a landscape via numerical integration. However, this procedure can be extremely computationally expensive for multidimensional collective variable (CV) spaces. Therefore, many free-energy calculations are restricted to only a few dimensions.
To tackle this problem, we introduce a method combining Bayesian quadrature with umbrella integration. Bayesian quadrature is a numerical method for estimating the value of integrals based on Bayesian inference. Since umbrella sampling gives us a free-energy gradient estimation, we can use Bayesian quadrature directly to calculate the integrand, i.e., the free energy. Bayesian quadrature performs the expensive calculations on the most informative samples of the free-energy landscape, which are iteratively selected based on a probabilistic model.
Moreover, the approach can also search for low free-energy regions, i.e., optimal transition pathways. In the talk, I will present this method applied to analytical surfaces and peptide conformational changes. I will also provide an outlook for other potentially interesting systems.
Discovery of new salt hydrates for thermochemical energy storage: deep learning based on stoichiometry
Speaker: Alexander Korotkevich
Abstract:
More than 50% of energy consumed in buildings is for thermal end use. Hence, in view of the global energy crisis there is urgency to develop technologies that efficiently store and reuse the available heat. A promising approach addressing this challenge is based on hydration reactions of solid salts:
Salt• mH2O + (n-m)H2O(g)↔ Salt• nH2O + Q
This process is reversible, hydration reaction leads to release of heat, while the dehydration is endothermic. reversible hydration reactions Such “heat batteries” have high energy densities (>1GJ/m3) and are environmentally benign. However, these materials need improvement in durability and operational conditions for real-life applications. We aim to discover new promising salt hydrates and optimize their design. This design space is however quite large (> 10^8 reactions), making the conventional high-throughput screening unreasonable.
To approach this problem, we apply artificial intelligence. We fit machine learning models on thermochemical data for salt hydration reactions. As model input, we use reaction representations based on stoichiometry. Those simple representations are available for any hydration reaction, however they encode only limited information about the reactants. We tackle this by introducing chemical contextual awareness, similar to context attention in natural language processing. Thanks to the attention mechanism we can learn information-rich graph-like representations of salt hydrates directly from data. This way we developed models capable of predicting heat and energy density of hydration reactions with accuracies.
This seminar will take place in room C4.174