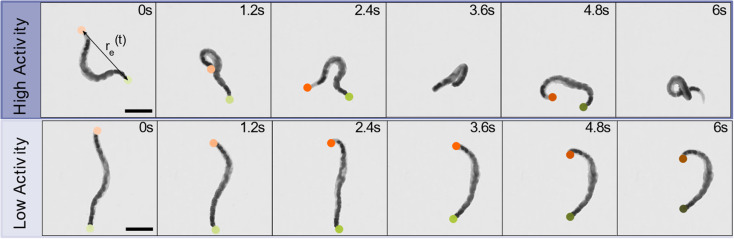
Data-driven approach to develop active filament model for self-propelled worms
Recently, living worms such as T. tubifex and California blackworms have gained attention as experimental model systems for self-propelled active filaments [1,2]. Early studies suggest these worms can be modeled by physically motivated active polymer models, where filaments are driven by tangential forces along their backbone, enabling self-propulsion. However, our recent findings indicate that such models capture worm dynamics only qualitatively [3]. In more complex environments, data-driven models offer the potential to capture their intricate dynamics more accurately.
In this internship, you will use data-driven approaches to develop more realistic models of worm dynamics, allowing for time- and space-dependent active forces as well as transverse force fluctuations. The novel model developed through this data-driven approach can inform the design of biomimetic robotic worms with enhanced functionalities. This project involves using the high-performance molecular dynamics software Hoomd-blue, along with code development for data analysis and AI algorithms. The work will be done in collaboration with Antoine Deblais (worm dynamics) and Alberto Perez de Alba Ortiz (machine learning).
References
- A. Deblais, A. C. Maggs, D. Bonn, and S. Woutersen. Phase separation by entanglement of active polymerlike worms. Phys. Rev. Lett., 124:208006 (2020)
- Chantal Nguyen, Yasemin Ozkan-Aydin, Harry Tuazon, Daniel. I. Goldman, M. Saad Bhamla, and Orit Peleg. Emergent collective locomotion in an active polymer model of entangled worm blobs. Frontiers in Physics, 9:540 (2021).
- Locomotion of Polymerlike Worms in Porous Media R. Sinaasappel, M. Fazelzadeh, T. Hooijschuur, S. Jabbari-Farouji, and A. Deblais, arXiv:2407.18805 (2024).
Contact
Dr. Sara Jabbari-Farouji s.jabbarifarouji@uva.nl at University of Amsterdam. You will be working in interdisciplinary computational soft matter lab.