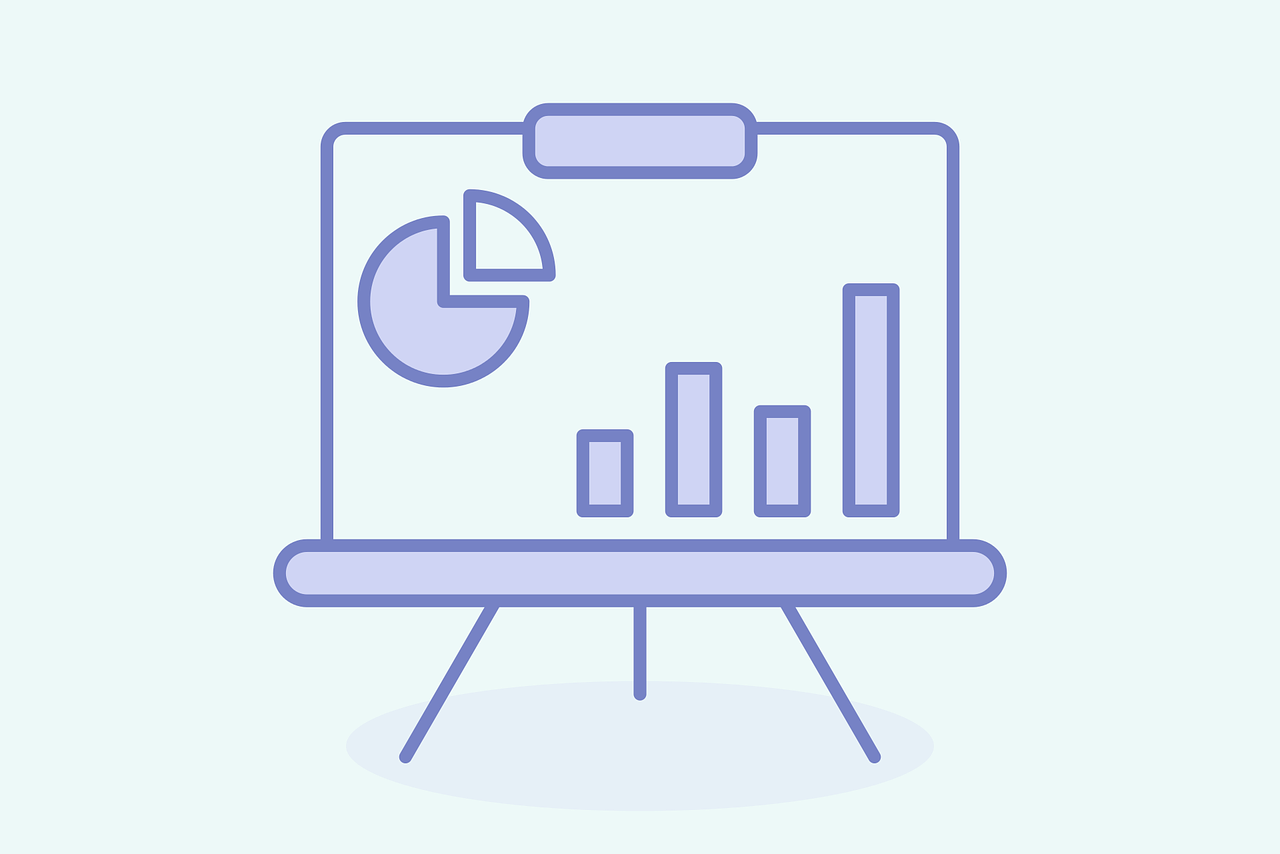
Learning without neurons in physical systems
Speaker: Menachem Stern (AMOLF)
Abstract:
From electrically responsive neuronal networks to immune repertoires, biological systems can learn to perform complex tasks. In this seminar, we explore physical learning, a framework inspired by computational learning theory and biological systems, where networks physically adapt to applied forces to adopt desired functions. Unlike traditional engineering approaches or artificial intelligence, physical learning is facilitated by physically realizable learning rules, requiring only local responses and no explicit information about the desired functionality. Our research shows that such local learning rules can be derived for broad classes of physical networks and that physical learning is indeed physically realizable, without computer aid, through laboratory experiments. Furthermore, we demonstrate that learning induces architectural changes in the physical network, leading to a decrease in the effective physical dimension and a realignment of its inherent coordinate system to the learned task. These effects suggest a method for discovering the task that a novel network may have been trained for. By leveraging the advances of statistical learning theory in physical machines, we propose physical learning as a promising bridge between computational machine learning and biology, with the potential to enable the development of new classes of smart metamaterials that adapt in-situ to users’ needs.
This seminar will take place in room C4.174