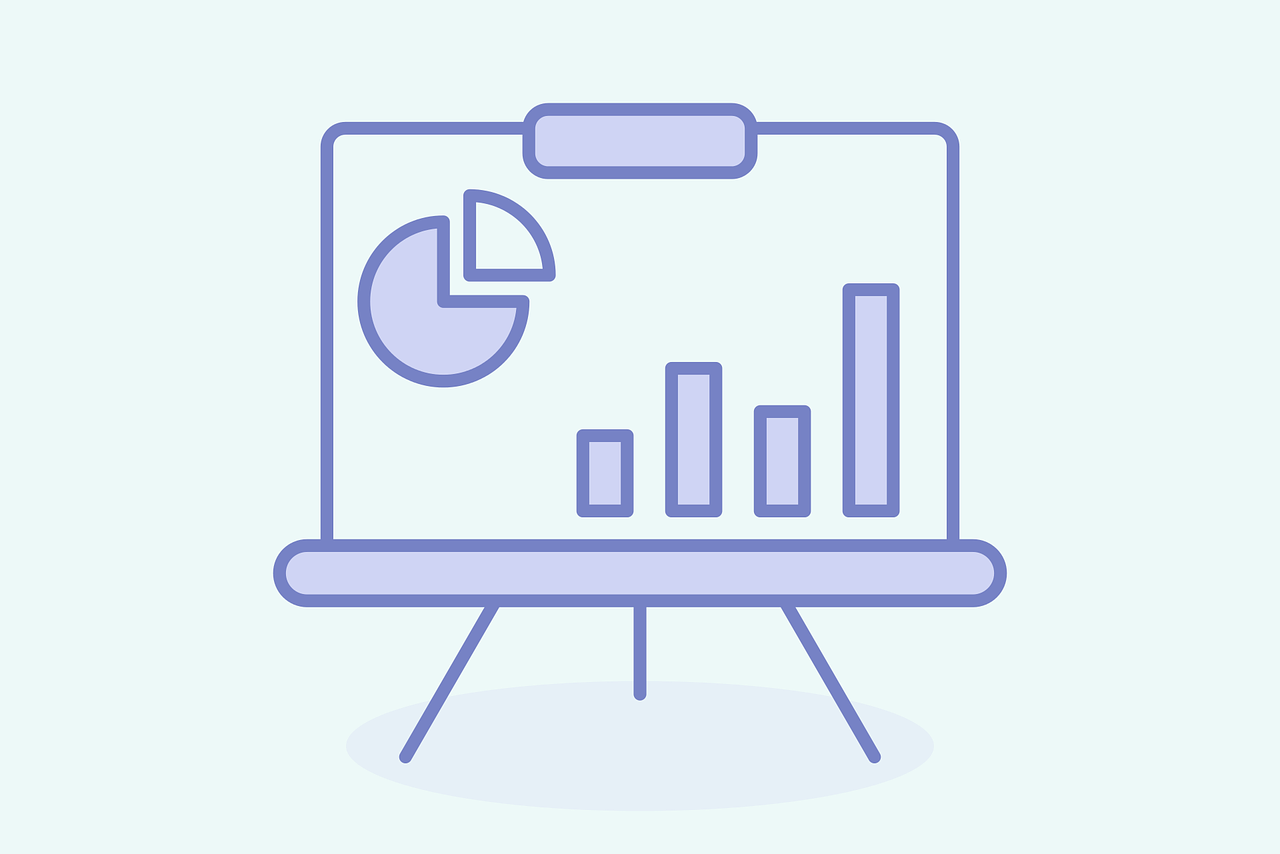
Title: Predicting molecular properties and designing new molecules through molecular machine learning
Speaker: Jana Marie Weber(TU Delft)
Abstract:
The discovery and development of novel functional molecules impacts the sustainability transition through a multitude of aspects, e.g. by discovering improved (bio)catalysts, novel energy materials, biodegradable products, or more sustainable reaction pathways. Communities from computational chemistry, material design, and bioinformatics are jointly supporting such endeavours through the development of machine learning algorithms on molecular data: molecular machine learning. In this talk, I will highlight selected building blocks of molecular machine learning approaches: finding a suitable molecular representation, developing molecular property predictors, and strategies for inverse molecular design. We will see recent machine learning concepts on a range of molecular data. I will talk about self-supervised learning on state-of-the art graph representations [1,2], combinations of rule-based and deep learning methods [3], and generative AI guided by molecular properties [4,5,6]. As molecular systems, we will cover copolymers, proteins, and chemical reactions.
References
1: Gao, Q., Dukker, T., Schweidtmann, A. M., & Weber, J. M. (2024). Self-supervised graph neural networks for polymer property prediction. Molecular Systems Design & Engineering.
2: Aldeghi, M., & Coley, C. W. (2022). A graph representation of molecular ensembles for polymer property prediction. Chemical Science, 13(35), 10486-10498.
3: van Wijngaarden, M., Vogel, G., & Weber, J. M. (2024). Completing Partial Reaction Equations with Rule and Language Model-based Methods. In Computer Aided Chemical Engineering (Vol. 53, pp. 3139-3144). Elsevier.
4: Vogel, G., Sortino, P., & Weber, J. M. Graph-to-String Variational Autoencoder for Synthetic Polymer Design. In AI for Accelerated Materials Design-NeurIPS 2023 Workshop.
5: Villegas-Morcillo, A., Weber, J. M., & Reinders, M. J. (2023). Guiding diffusion models for antibody sequence and structure co-design with developability properties. bioRxiv, 2023-11.
6: Luo, S., Su, Y., Peng, X., Wang, S., Peng, J., & Ma, J. (2022). Antigen-specific antibody design and optimization with diffusion-based generative models for protein structures. Advances in Neural Information Processing Systems, 35, 9754-9767.
Short bio
Jana M. Weber is an assistant professor for artificial intelligence in bioscience at TU Delft in the Department of Intelligent Systems since 2022. There, she manages the AI4b.io lab and she is part of the Delft Bioinformatics Lab. In 2022, Jana defended her PhD in Chemical Engineering from the University of Cambridge for her work on circular chemistry through large scale reaction network analysis and optimization. Prior to that, she obtained her MSc in Environmental (Process) Engineering from RWTH Aachen University with her Master’s thesis performed at the Jülich Research centre at the institute of bio- and geosciences. Jana’s research interests cover molecular machine learning and network science for a broad range of environmental applications.
This seminar will take place in room C4.174