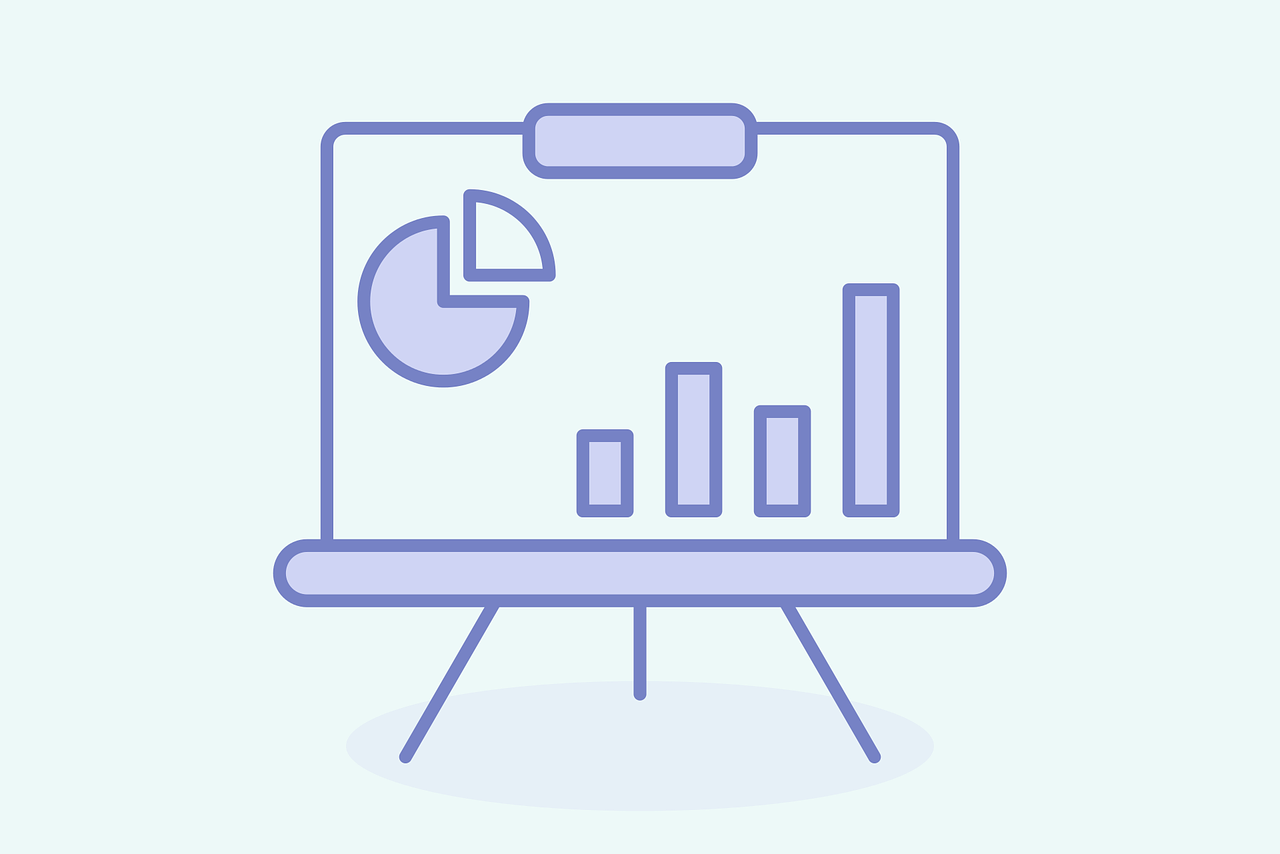
Title: Combining data-driven and physics-based approaches to predict, understand, and control active matter dynamics
Speaker: Mike Hagan (Brandeis University)
Abstract:
Active matter is composed of particles that generate forces, which leads to spectacular emergent dynamics resembling the lifelike properties of biological organisms. Yet, active materials exhibit diverse dynamical states, most of which have chaotic dynamics that do not produce work or other functions. Designing or controlling an active material to select a state corresponding to a desirable function requires accurate dynamical models. However, developing models using traditional statistical physics approaches is challenging because active materials lack the scale separation characteristic of equilibrium systems.
In this presentation, I will discuss efforts to use data-driven techniques to address this challenge in the context of a model active material, microtubule-based active nematics. I will describe two complementary approaches. In the first, we have adapted a method to discover optimal continuum models directly from spatiotemporal data, using sparse regression. We have identified several approaches to mitigate measurement errors in the data. We find that the method can reveal the relative contributions of different physical mechanisms, and quantitatively estimates key experimental parameters. In the second approach, we have used deep learning to automatically learn and forecast active nematics dynamics, using data from particle simulations and experiments. We find that the method can predict spatiotemporal dynamics including the spontaneous creation and annihilation of defects, but that inaccuracies arise from measurement errors in this complex system. Further, I will discuss reduced-dimensional representations of the forecaster, which reduce training time and may facilitate human interpretation. In the second approach, we have adapted a method to discover optimal continuum models directly from spatiotemporal data, using sparse regression. We have identified several approaches to mitigate measurement errors in the data. We find that the method can reveal the relative contributions of different physical mechanisms, and quantitatively estimates key experimental parameters, e.g. how the ‘activity’ depends on ATP concentration. I will also describe a tensor-based formulation of the method for 3D systems, and its application to 3D simulations of dry active nematics.
Time permitting, I will discuss how models discovered by these approaches can be combined with control theory to drive active materials toward particular emergent behaviors.
This seminar will take place in room B1.25