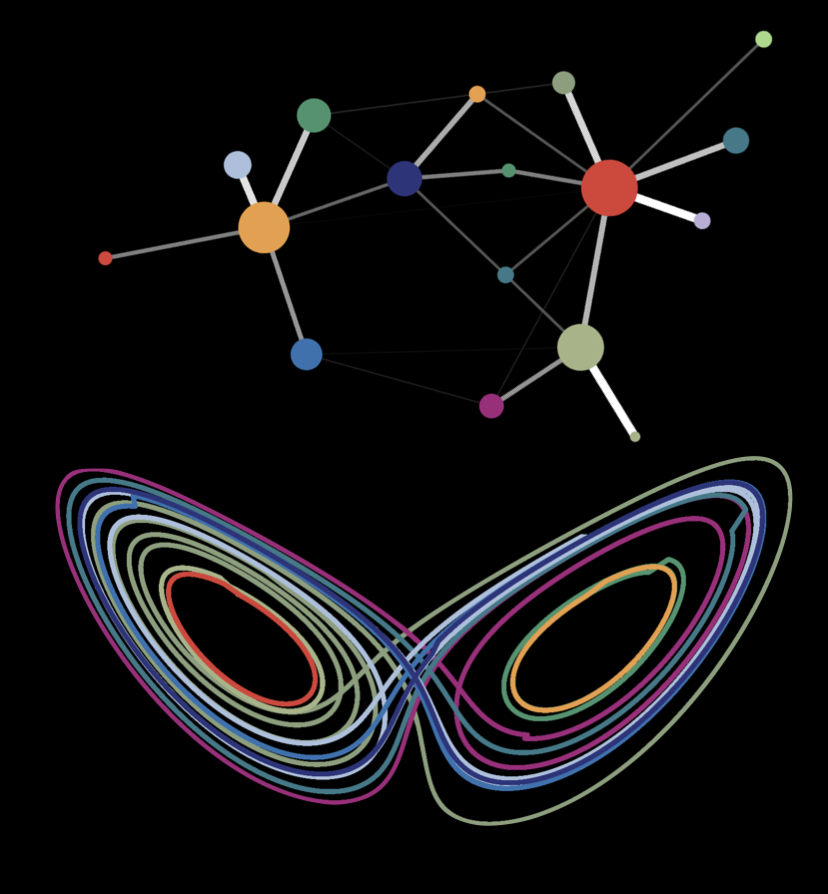
Title: Orbit hierarchies determine the long-term predictability of chaotic systems
Speaker: William Gilpin, Department of Physics (University of Texas)
Abstract:
The striking fractal geometry of strange attractors underscores the generative nature of chaos: like probability distributions, repeated measurements of chaotic systems produce arbitrarily-detailed information about the underlying attractor. Chaotic systems thus pose a unique challenge to modern statistical forecasting models, requiring representations that correctly encode their fractal geometry while also capturing their underlying mathematical properties. I will describe my recent work on representing and forecasting chaotic systems. Using a collection comprising hundreds of known chaotic dynamical systems spanning fields such as astrophysics, climatology, and biochemistry, I show that chaoticity and empirical predictability are only weakly correlated. Instead, the performance of contemporary machine learning algorithms is limited by topological properties of the underlying dynamical systems, which execute transitions among sets of unstable periodic orbits. I will show how tools from chaos can assist in general statistical learning problems, such as time series classification, importance sampling, and symbolic regression.
This event will take place in room L1.08