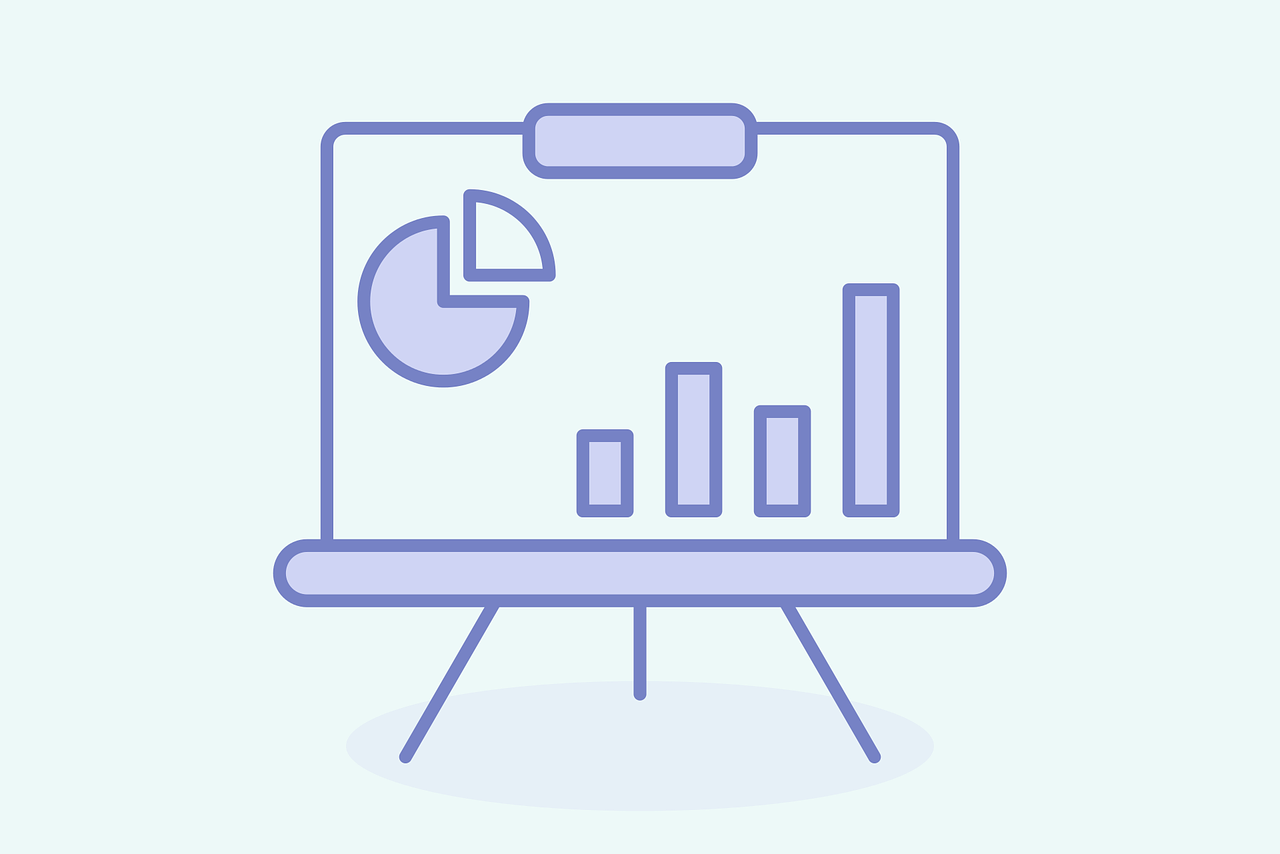
Title: Rational discovery of cardiolipin-selective small molecules by coarse-grained high-throughput simulations
Speaker: Bernadette Mohr (HIMS & IVI)
Abstract:
Subtle abnormalities in the lipid composition of mitochondrial membranes can have a profound impact on mitochondrial function. The inner mitochondrial membrane uniquely contains the phospholipid cardiolipin, which has been demonstrated to act as a biomarker for a number of diverse pathologies. Here we present a data-driven approach that combines a deep learning-enabled active learning workflow with coarse-grained molecular dynamics simulations and alchemical free energy calculations to discover compounds able to selectively permeate into cardiolipin-containing membranes. By employing transferable coarse-grained models we efficiently navigate the all-atom design space corresponding to small organic molecules with molecular weight less than ~500 Da. After direct simulation of only 0.42% of our coarse-grained search space we identify molecules with considerably increased levels of cardiolipin selectivity compared to a widely used cardiolipin probe. Our accumulated simulation data enables us to derive interpretable design rules linking coarse-grained structure to cardiolipin selectivity. These design rules are corroborated by fluorescence anisotropy measurements of two conforming compounds. Our findings highlight the potential of coarse-grained representations and multiscale modelling for materials discovery and design.
Title: Machine Learning Zero Modes in Combinatorial Metamaterials
Speaker: Ryan van Mastrigt (IoP)
Abstract:
All around us, simple building blocks combine into complex systems with emergent properties. A general problem is to classify these properties into distinct classes. Statistical measures often play an important role, yet for many cases, the misalignment or perturbation of a single building block can have a dramatic effect on the collective properties. Here we show that Convolutional Neural Networks (CNNs) are able to accurately classify the design space of a combinatorial metamaterial, despite its sensitivity to small perturbations and the sparsity of the data set. Moreover, we show that in spite of the combinatorial explosion of the design space, the trained networks’ accuracy remains high over a range of increasing design sizes. Together, our results show that neural networks are an excellent tool for combinatorial classification problems with complex underlying rules.