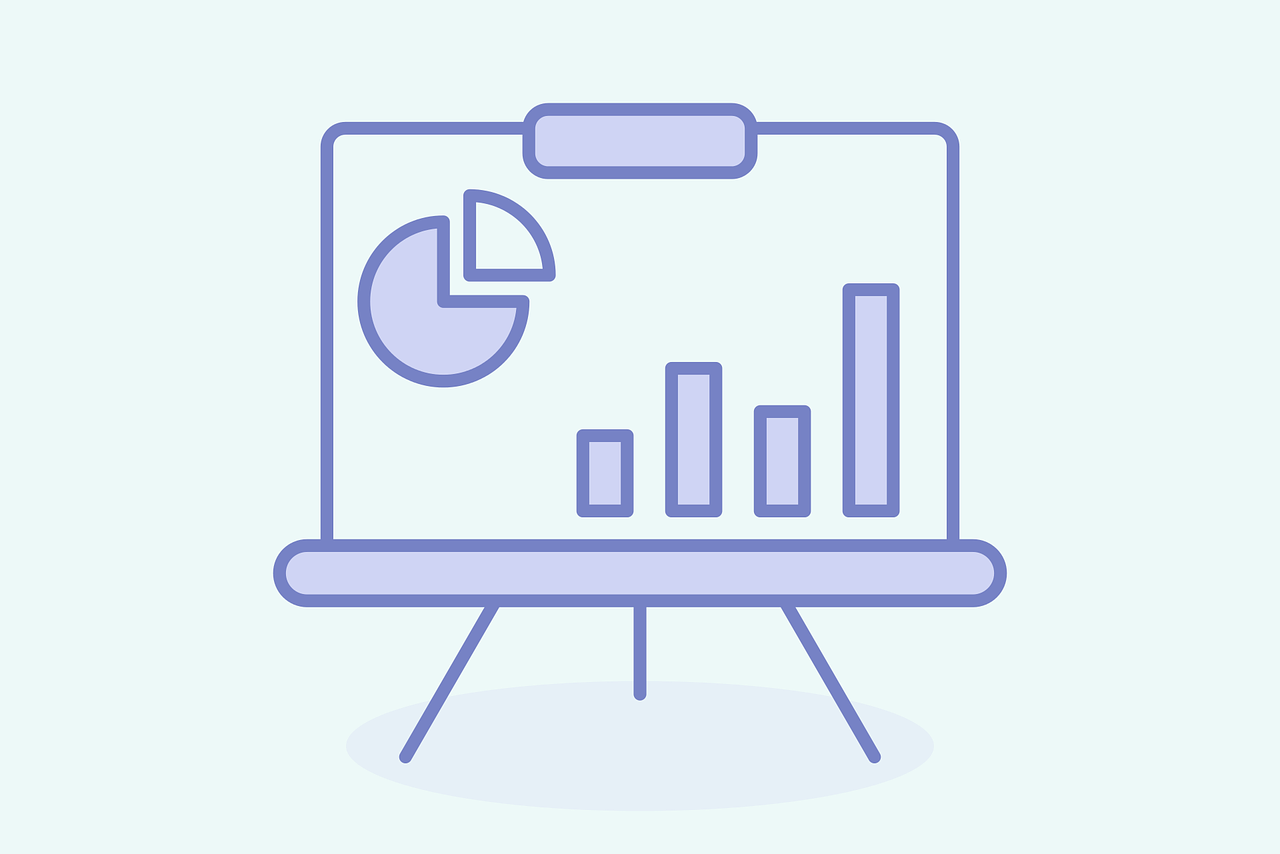
Title: Maximally-predictive, coarse-grained dynamics from data
Speaker: Greg Stephens, Free University of Amsterdam
Date: Tuesday 8th September, 2020 at 14:00
Abstract: Complex systems generally exhibit dynamics with fine-scale unpredictability yet larger-scale order. We propose to capture these features through the principled extraction of coarse-grained, ensemble dynamics from incomplete measurements, a generic condition when sampling from data. We reconstruct a state space by concatenating measurements in time, partitioning the resulting sequences, and choosing the sequence length to minimize the entropy rate, thus maximizing prediction. The entropy rate, a fundamental measure of dynamical complexity, is estimated through a Markov approximation of the state space dynamics and corresponds to the Kolmogorov-Sinai entropy when applied to deterministic dynamical systems. Within this partitioned space, we capture the dynamics of densities through transfer operators; non-linear dynamics are thus subsumed into linear, ensemble evolution, yielding a simple, yet accurate, model on multiple scales. Applicable to both deterministic and stochastic systems, we illustrate our approach in the Langevin dynamics of a particle in a double-well potential and the Lorenz system. We discuss applications of these ideas to systems in which the fundamental dynamics are unknown.
Events info sent to the CSM mailing list