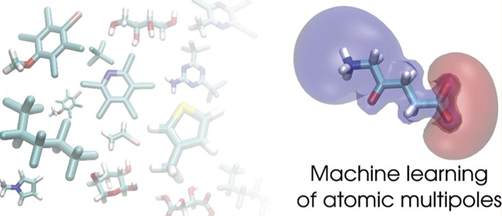
The modeling of real materials, such as organic light emitting diodes and solar cells is inherently multiscale. For example, understanding interconversion of electrons, holes, and excitons requires a high resolution model, incorporating both the relevant physics and chemically-specific parameters. Polarizable force fields are ideally suited for this purpose. Unfortunately, their parametrization requires extensive manual tuning, at odds with a screening strategy that is desirable to design compounds with optimized properties.
Machine learning of multipole electrostatic parameters
The student will be involved in further developing efforts at the use of kernel-based machine learning (ML) methodologies to automate and ease parametrization for advanced force fields of organic molecules. He/she will be responsible for the use of covariant kernels for the learning of multipole electrostatic parameters across the chemical space of organic molecules. Previous work in this direction can be found in [1], [2], and [3]
[1] https://doi.org/10.1021/acs.jctc.5b00301 [2] https://doi.org/10.1063/1.5009502@jcp.2018.DETC2018.issue-1 [3] https://doi.org/10.1021/acs.jctc.9b01256
We will extend this approach to the learning of multipole parameters for not only neutral organic molecules, but also anionic and cationic compounds. The student is expected to gain experience in building databases of electronic properties by means of high-throughput quantum-chemical calculations and training covariant kernels for physical properties.
Contact: Dr. Tristan Bereau (t.bereau@uva.nl) Dr. Denis Andrienko (andrienk@mpip-mainz.mpg.de)